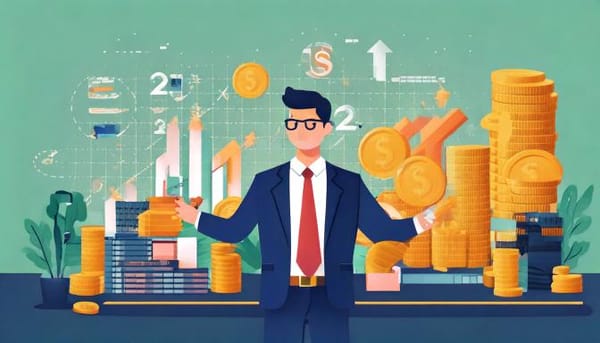
Finance
Some `options` for 2025
Alphabet today has a lower valuation and more diversified revenue streams compared to Nvidia’s more concentrated revenue streams in data centers optimized for AI.
Product
HTTP/2’s primary performance advantage lies in its multiplexing feature, where multiple streams are handled simultaneously over a single TCP connection. Inefficient multiplexing can lead to inefficiencies. It's important to use the right tools and strategy for HTTP/2 applications.
Product
Monitoring solutions typically involve the collection of logs, traces, and metrics that assist organizations in comprehending the behavior of their applications. These tools process vast quantities of data to provide valuable insights. Traditionally, monitoring was conducted in silos, necessitating separate tools for monitoring various aspects, such as applications, networks, security,
AI
The last couple of week saw all of the AI related gains drop at a steep rate. It’s earnings season and looks like Wall St. does not like it when billions of $ of investment do not give you the return they expected to see. META, Google and Microsoft earnings
Apple
Apple
Apple’s ability to observe technology and maker maturation before building or integrating it in their ecosystem has paid off in the long run. AI is no different.
Artificial Intelligence
Nvidia's market value has exceeded Tesla & Amazon COMBINED!
AI
In case you missed it, the video is available to watch again: rabbit r1 - pickup party nyc live stream nowtune in and join the partypickup party nyc live stream now I ordered one back in January. I haven’t gotten a shipping notice yet. However, there is a lot
App
We all have ideas that we would like to build and see them flourish. But thats the things about ideas, they aren't always good. The little owl helps you keep track of your good ideas.
AI
In today's context, Humane's AI pin is probably the first device where I see personal computing heading towards.
Random Ramblings from a Product Manager, Photographer and Food enthusiast.
One of the most important announcement at the scary fast event came at the end.
Ideas are cheap. They come up all the time and end up in the backlog. Remember, really important ideas almost always come back.
If you have a blog, website, trying to sell online or have a small business - basically if you have an onine presence - a website; do not underestimate the power of Google search. Today, when you have to look for a service, a place to eat or literally anything
Ben Thompson on Stratechery Threads and the Social/Communications MapUnderstanding Threads and its threat to Twitter means understanding the current landscape of social media.Stratechery by Ben ThompsonBen Thompson Instagram’s Evolution has shown that this shift is possible, but the shift has been systemic and gradual — and even then
I have been away for a few weeks, traveling and attending conferences. While the conferences have been giving me an insight into the amazing things organizations are building (very heavily focused on AI/ML), I have been reading some fascinating articles during my down time. How to start a Go
I think I’m getting a hang of this. I still struggle with composition on macro as I continue to shoot the two ends of the spectrum; landscapes and macro.
Cade Metz for The New York Times: ‘The Godfather of A.I.’ Leaves Google and Warns of Danger Ahead On Monday, however, he officially joined a growing chorus of critics who say those companies are racing toward danger with their aggressive campaign to create products based on generative artificial intelligence,
It took me ~10 seconds to open the Apple's high-yield savings account today after answering ONE question and accepting the terms. It took me less than a second to set up my Apple Credit Cards cash back to go to my savings account. After playing around for about
Its the automation and the ecosystem is why I rely on my Apple Watch. I don't have to exclusively perform a task to keep tabs, it just does it for me.
LearnTheFlag has been doing well and I have been enjoying using it with my son to teach him about the different countries in the world. The latest addition of the Map view and including the Wiki link has been great. The Map view is pretty simple. The idea was to
Is Apple a Blessing, or a Curse in Disguise? Apple's convenience, ease of use and Ecosystem is what people are hooked on. How long would it be until you are also banking with Apple products?